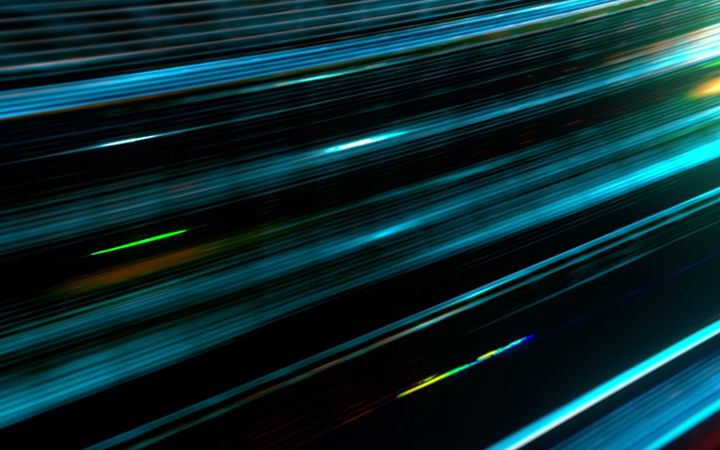
Safe and effective strategies for applying generative AI to your mission
Deciding whether to use artificial intelligence (AI) no longer seems the most important technology question for leaders and business strategists. It’s clear AI is here to stay so, today, the big question is “what AI model is best for my needs?”
Without a clear understanding of exactly what your AI solution should be doing, unleashing its power may seem less like a race to the finish line and more like a stop and go commute through heavy traffic. With motivation in the driver’s seat but cost riding the brake, AI projects can sometimes end up on a bumpy, winding road to development.
As you head down the AI path, a wise practice is to first perform a requirements assessment. A basic chatbot (or virtual assistant), for example, can only perform a limited number of static input-output tasks while a generative AI can take over a conversation, continuously improve, and require far less training oversight from humans. In performing your assessment, it’s equally important to also weigh the costs of mitigating risks that generative AI can introduce just as carefully as the cost of tools and development.
Generative AI means just that—that it generates something new based on a pattern it’s observed from the data it was provided. The larger the data set, the greater variety of content the AI can generate with confidence. However, when the AI lacks confidence in its data set, that’s when you can run into trouble with generative content.
Unlike traditional AIs, a generative AI can’t say “I don’t know” so it must fulfill the request. If that request is out of scope of its language model, the generative AI may struggle to force patterns where none exist. The challenge then becomes: When you can ask an AI any question in the world, how do you keep both it and your users on track to fulfilling the purpose you set for it?
Finding the AI model to suit your needs
If you’ve been anywhere near a news article this year, you may have read about flashy AI apps such as ChatGPT, which uses a large language model (LLM) to showcase its ability to learn and converse on its own.
An LLM is a neural network that contains billions of parameters and uses deep learning algorithms to mimic human understanding and teach itself. It can be trained on a massive quantity of unlabeled data, which means that a human hasn't had to sort and label that data first—a significant advantage over other models.
There are also different sizes of language model to consider. Smaller than the generalist LLM, a fine-tuned language model takes a model that has already been pre-trained for a given task and adjusts its training to make it a more effective specialist. Even smaller still is an edge language model which has kept its data set purposely small to grant the most control and quickest response times.
For our clients, supervised training—sometimes called human reinforcement learning—of a smaller specialist model provides the reassurance that every single piece of AI training data has been vetted by a human. Edge solutions in particular also tend to be less expensive, faster to run, and they can function offline if they're downloaded into an app. This can be critical when the rapid response time of the AI is the highest priority. A good example of edge AI is the Tesla self-driving car.
Human-reinforced AI often appeals to federal clients or any sector in which it is critically important to have 100% control over both response content and user-generated data. With a restricted language model, the range and flexibility of responses is limited but there is the assurance that nothing goes out to the internet that could train other open AI models. A self-supervised generative AI, on the other hand, has user-generated data in its training. The risk for some organizations is twofold: that these responses, based on observations of user data, are not fact-checked and that there is no way to guarantee that user data is protected if it’s been incorporated into the LLM.
Using generative AI to help a federal agency improve program delivery
Our client, HIV.gov, needed a solution that could use public health language that is very tightly controlled by the Centers for Disease Control and Prevention (CDC). Their AI solution needed to use a specific vocabulary of medical terms in a consistent way and follow CDC's phrasing verbatim, which changes often based on infectious disease research. To accomplish this, we started with a pre-trained edge model with a human reviewing and tagging every piece of training in the data set. Using human reinforcement learning ensured that user data was kept anonymous and private, which was a huge consideration for the HIV / AIDS community.
In working innovatively with HIV.gov, we used an LLM to help build upon this edge model without exposing user data—in essence making the LLM a brain and not a mouthpiece by leveraging its understanding without the risk of putting un-cited generative content in front of users. When we talk about risks with LLMs, the risk is typically only with the generative aspect and not with the many other functions where an LLM can substantially and positively influence development. When a generative model lacks the data to provide a confident answer, it will sometimes “hallucinate” to fill in the gaps and authoritatively cite incorrect or completely fabricated information as if it were fact. The inverse—too much irrelevant data—can also introduce algorithmic bias, which can create an unfair advantage to an arbitrary group of users.
That doesn’t mean we ignore all the benefits that generative AI can bring. Part of our digital modernization solution for HIV.gov leverages multiple other LLMs behind the scenes to help process analytics, improve content, build on existing training data, and detect patterns in user behavior that inform development decisions. The HIV.gov chatbot’s core purpose is to be a trustworthy source of critical HIV information and linkage to care. At every step of the way, we’ve ensured that there are no shortcuts that may compromise that trust and quality of content.
Establishing trust is not just important for federal clients with sensitive data and strictly controlled language like HIV.gov—all solutions with AI technology are under more scrutiny than ever before. Quality of content, generative or not, is an essential element for leaders and strategists to consider. Before beginning development, making an informed strategy about the balance of responsibilities between humans and AIs helps to build a robust solution that garners both success and credibility with far fewer speed bumps along the way.